Overview
Biodiversity is crucial for ecosystem stability and resilience, acting as a natural defense against disturbances like climate change and invasive species. It also supports the economy by providing essential resources such as food, medicine, and genetic material. Understanding biodiversity is key for sustainable resource management, ensuring these resources remain available for future generations. BIOSCAN’s global biodiversity assessment aims to comprehensively catalog living organisms worldwide, encompassing the intricate tapestry of insect biodiversity. As a fundamental component of global ecosystems, insects contribute significantly to pollination, nutrient cycling, and overall ecosystem stability, embodying a remarkable diversity of species.
BIOSCAN-5M

A comprehensive dataset containing multi-modal information for over 5 million insect specimens, and it significantly expands existing image-based biological datasets by including taxonomic labels, raw nucleotide barcode sequences, assigned barcode index numbers, and geographical information. Every record has both image and DNA data.
Attributes
Each record of the BIOSCAN-5M dataset contains six primary attributes:
- RGB image
- DNA nucleotide barcode sequence
- Barcode Index Number (BIN)
- Biological taxonomic classification
- Geographical information
- Specimen size
Benchmark Experiments
BIOSCAN-5M paper proposes three benchmark experiments to demonstrate the impact of the multi-modal data types on the classification and clustering accuracy:
- We pretrain a masked language model on the DNA barcode sequences of the BIOSCAN-5M dataset and demonstrate the impact of using this large reference library on species- and genus-level classification performance.
- We propose a zero-shot transfer learning task applied to images and DNA barcodes to cluster feature embeddings obtained from self-supervised learning, to investigate whether meaningful clusters can be derived from these representation embeddings.
- We benchmark multi-modality by performing contrastive learning on DNA barcodes, image data, and taxonomic information. This yields a general shared embedding space enabling taxonomic classification using multiple types of information and modalities.
Dataset Sources
Please use the following links to access the dataset packages and updates:
- Website: https://biodiversitygenomics.net/5M-insects/
- GitHub: https://github.com/zahrag/BIOSCAN-5M
- Google Drive: https://drive.google.com/drive/u/1/folders/1Jc57eKkeiYrnUBc9WlIp-ZS_L1bVlT-0
- Hugging Face: https://huggingface.co/datasets/Gharaee/BIOSCAN-5M
- Zenodo: https://zenodo.org/records/11973457
- Kaggle: https://www.kaggle.com/datasets/zahragharaee/bioscan-5m
- Paper: https://arxiv.org/abs/2406.12723
Citation
If you make use of the BIOSCAN-5M dataset and/or its code repository, please cite the following paper:
@misc{gharaee2024bioscan5m,
title={{BIOSCAN-5M}: A Multimodal Dataset for Insect Biodiversity},
author={Zahra Gharaee and Scott C. Lowe and ZeMing Gong and Pablo Millan Arias
and Nicholas Pellegrino and Austin T. Wang and Joakim Bruslund Haurum
and Iuliia Zarubiieva and Lila Kari and Dirk Steinke and Graham W. Taylor and Paul Fieguth and Angel X. Chang},
year={2024},
eprint={2406.12723},
archivePrefix={arXiv},
primaryClass={cs.LG},
doi={10.48550/arxiv.2406.12723}}
BIOSCAN-1M
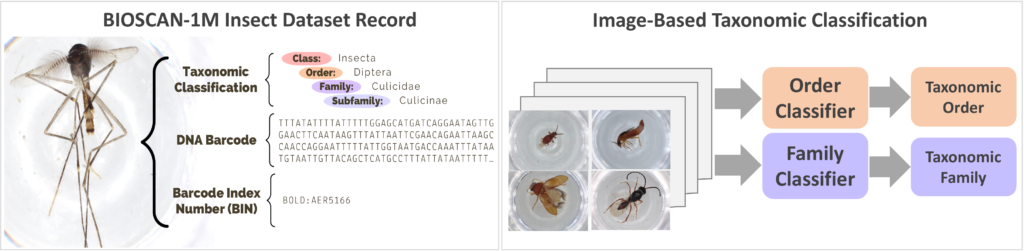
In 2023, we proposed a rich repository featuring one million intricately labeled insect images. Each entry underwent expert taxonomic classification and was enriched with genetic data, including raw nucleotide barcode sequences and barcode index numbers—a genetic-based proxy for species classification. The BIOSCAN-1M Insect Dataset is designed primarily to support the training of advanced computer-vision models for image-based taxonomic assessments. Apart from its application in machine learning, it distinguishes itself with unique attributes including distinctive long-tailed class-imbalance distribution commonly observed in biological datasets. The taxonomic labeling adopts a hierarchical classification scheme, presenting a nuanced and fine-grained challenge, particularly at finer-grained taxonomic levels. This distinctive feature not only advances machine learning but also extends its relevance to the broader machine learning community. The initial phase of the project has been successfully completed with the release of the BIOSCAN-1M Insect Dataset, featured in the Advances in Neural Information Processing Systems (NeurIPS 2023) Datasets & Benchmarks Track.
Attributes
Each record of the BIOSCAN-1M Insect dataset contains 4 primary attributes:
- RGB Image
- DNA nucleotide barcode sequence
- Barcode Index Number (BIN)
- Biological taxonomic classification
Benchmark Experiments
BIOSCAN-1M Insect paper proposes two benchmark experiments on three subsets of the dataset:
- Image-based taxonomic classification on 16 distinct taxonomic orders within the insect community.
- Image-based taxonomic classification on 40 distinct taxonomic families within the insect community.
Dataset Sources
Please use the following links to access the dataset packages and updates:
- Website: https://biodiversitygenomics.net/projects/1m-insects/
- GitHub: https://github.com/zahrag/BIOSCAN-1M
- Google Drive: https://drive.google.com/drive/u/1/folders/1kD9cXuQ1FdL30etp7sjy_Gs_NAAJ3EXI
- Zenodo: https://zenodo.org/records/8030065
- Kaggle: https://www.kaggle.com/datasets/zahragharaee/bioscan-1m-insect-dataset
- Hugging Face: https://huggingface.co/datasets/Gharaee/BIOSCAN_1M_Insect_Dataset
- Paper: https://arxiv.org/abs/2307.10455
Citation
If you make use of the BIOSCAN-1M Insect dataset and/or its code repository, please cite the following paper:
@inproceedings{gharaee2023step,
title={A Step Towards Worldwide Biodiversity Assessment: The {BIOSCAN-1M} Insect Dataset},
booktitle={Advances in Neural Information Processing Systems},
author={Gharaee, Z. and Gong, Z. and Pellegrino, N.
and Zarubiieva, I. and Haurum, J. B. and Lowe, S. C. and McKeown, J. T. A. and Ho, C. Y
and McLeod, J. and Wei, Y. C. and Agda, J. and Ratnasingham, S. and Steinke, D. and Chang, A. X. and Taylor, G. W. and Fieguth, P.},
editor={A. Oh and T. Neumann and A. Globerson and K. Saenko and M. Hardt and S. Levine},
pages={43593--43619},
publisher={Curran Associates, Inc.},
year={2023},
volume={36},
url={https://proceedings.neurips.cc/paper_files/paper/2023/file/87dbbdc3a685a97ad28489a1d57c45c1-Paper-Datasets_and_Benchmarks.pdf},}
Directors
Post Docs
Students
Alumni
Related publications
Conference Papers