The systematic evaluation of synthetic aperture radar (SAR) data analysis tools, such as segmentation and classification algorithms for geographic information systems, is difficult given the unavailability of ground-truth data in most cases. Therefore, testing is typically limited to small sets of pseudo ground-truth data collected manually by trained experts, or primitive synthetic sets composed of simple geometries. In this work, we investigate the potential of employing an alternative approach, which involves the synthesis of SAR data and corresponding label fields from real SAR data for use as a reliable evaluation testbed.
Most previous methods are designed to generate textural characteristics only and cannot provide a corresponding label field. Moreover, in many situations, those texture methods are ill-suited and do not capture the complex structural characteristics found in remote sensing data.
In this work, we address the above issues by decoupling the synthesis of texture (Fig. 1) and label field (Fig. 2) into two different modeling structures. That is, we will explicitly synthesize the discrete-state label field (Fig. 3), which contains the complex structural characteristics of the remote sensing image, and separately synthesize the textures (Fig.4) of remote sensing images using a modification of the nonparametric texture synthesis strategy.

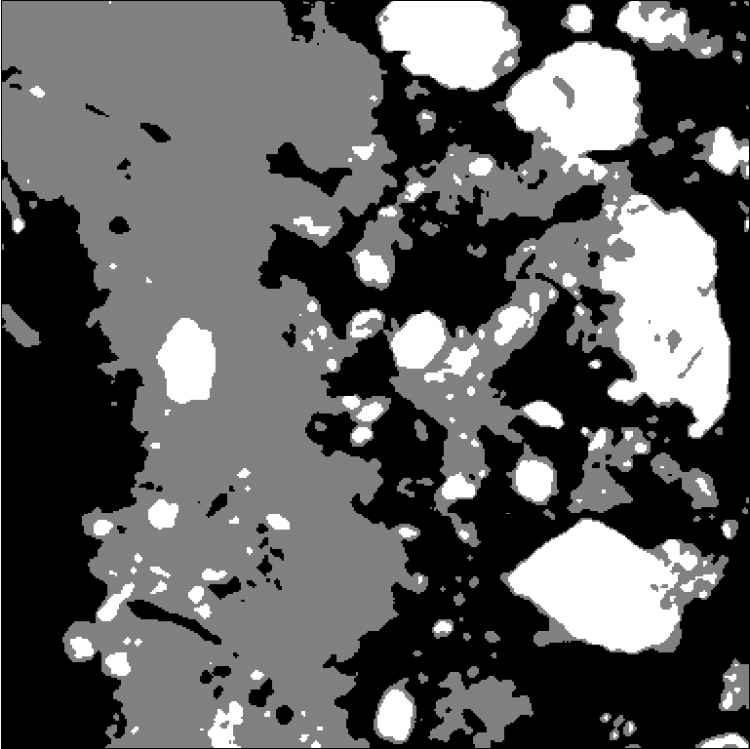

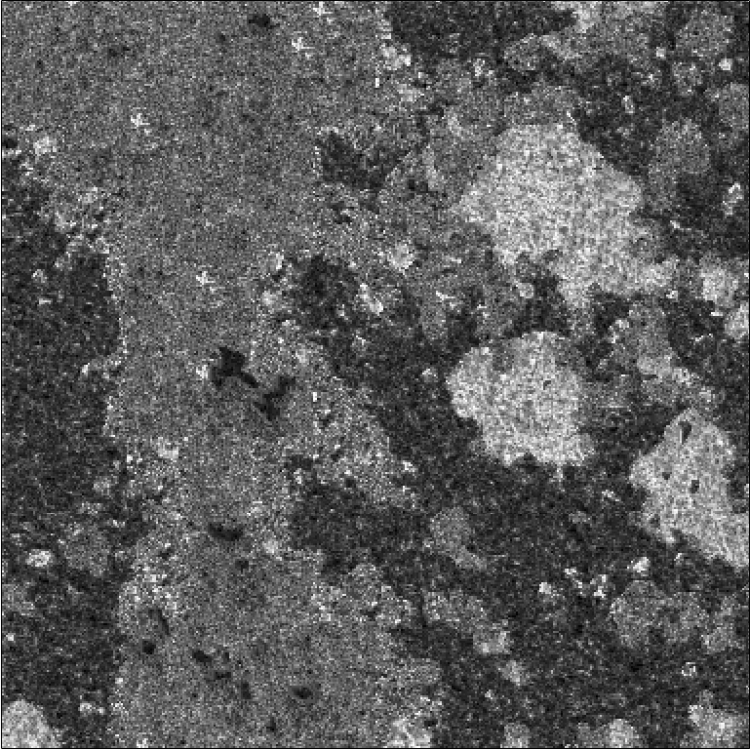
Given the scale-dependent, non-stationary nature of remotely sensed data, a new modeling approach that combines a resolution-oriented hierarchical method with a region-oriented binary tree structure is introduced to synthesize such complex discrete-state structures in a realistic manner. (Fig. 5)

Synthesis results using operational RADARSAT-1 SAR sea-ice imagery provided by the Canadian Ice Service (CIS) show that the proposed approach can well model complex nonstationary scale structures, thus making it well suited for synthesizing SAR data and the corresponding label fields for potential use in the systematic evaluation of SAR data analysis tools.
Related people
Directors
Students
Alumni
Related publications
Conference Papers
Conference Papers
Leigh, S., Z. Wang, and D. A. Clausi, “Evaluation of MAGIC Sea Ice Classifier on 61 Dual Polarization RADARSAT-2 Scenes”, IEEE Geoscience and Remote Sensing Symposium, Quebec City, Quebec, Canada, IEEE Geoscience and Remote Sensing Symposium, 2014. Get it here.